Queuing Theory Definition Elements And Example
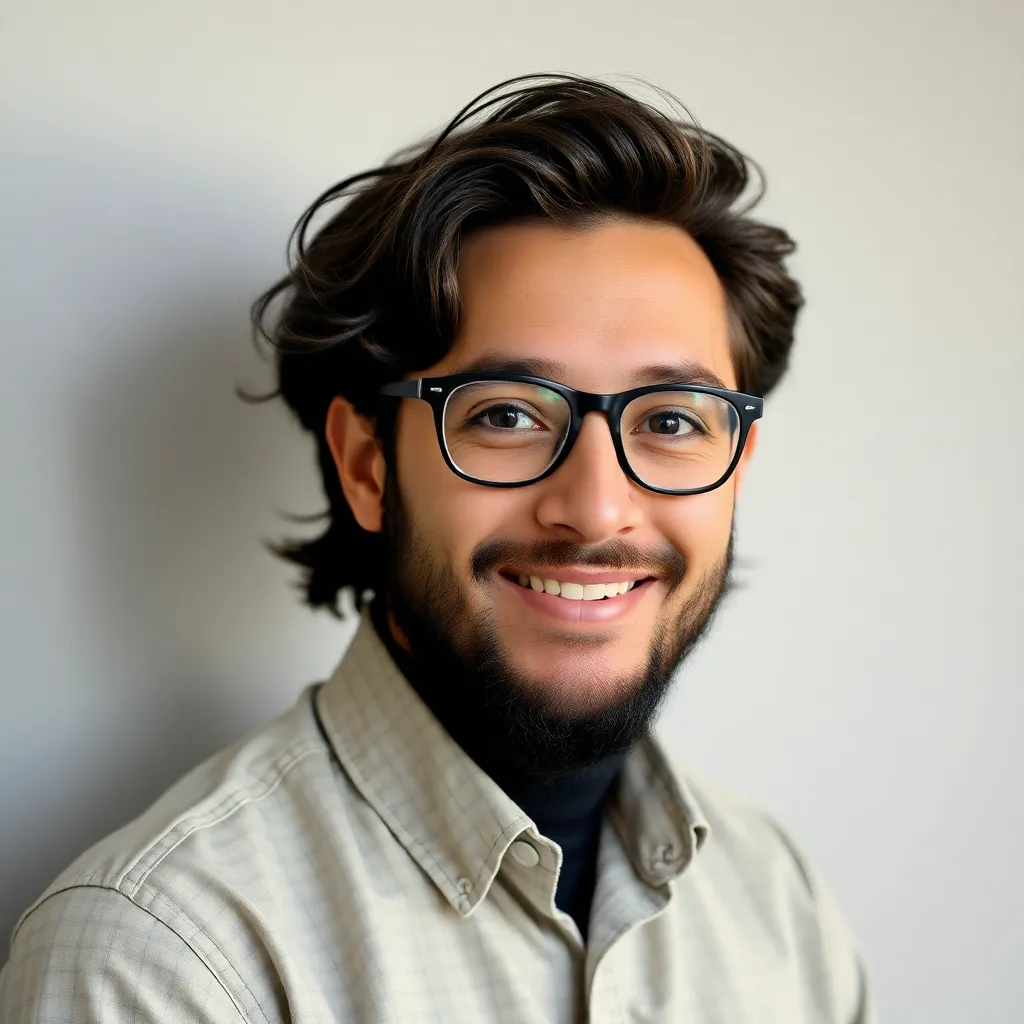
adminse
Mar 31, 2025 · 10 min read

Table of Contents
Unveiling the Secrets of Queuing Theory: Definitions, Elements, and Real-World Applications
What makes queuing theory so crucial for optimizing processes and enhancing efficiency?
Queuing theory is the bedrock of modern operational management, providing the tools to analyze, predict, and improve the performance of systems facing congestion.
Editor’s Note: This comprehensive guide to queuing theory has been published today.
Why Queuing Theory Matters
In today's fast-paced world, understanding and managing queues is paramount across numerous industries. From call centers and hospitals to manufacturing plants and airport security, scenarios involving waiting lines are ubiquitous. Queuing theory, a branch of mathematics and operations research, provides a rigorous framework for analyzing these waiting line situations. By understanding the underlying principles, organizations can optimize resource allocation, reduce waiting times, improve customer satisfaction, and ultimately, boost efficiency and profitability. This applies not just to tangible queues of people but also to virtual queues like network packets waiting for transmission or tasks waiting for processing in a computer system. The implications are far-reaching, impacting customer experience, operational costs, and overall system performance.
Overview of the Article
This article offers a comprehensive exploration of queuing theory, starting with fundamental definitions and progressing to essential elements like arrival rates, service rates, and queue disciplines. We'll delve into various queuing models, illustrate their applications with real-world examples, and discuss the limitations and extensions of this powerful analytical tool. Readers will gain a practical understanding of how queuing theory can be applied to improve the efficiency of various systems.
Research and Effort Behind the Insights
The insights presented in this article are drawn from a wide range of sources, including seminal works in operations research, contemporary research papers, and real-world case studies. The analysis incorporates mathematical models and statistical methods, ensuring accuracy and reliability. The aim is to provide readers with a robust and up-to-date understanding of queuing theory and its applications.
Key Concepts in Queuing Theory
Key Concept | Description |
---|---|
Arrival Rate (λ) | The average number of customers or jobs arriving at the system per unit of time. |
Service Rate (μ) | The average number of customers or jobs served per unit of time. |
Queue Length (L) | The average number of customers or jobs waiting in the queue. |
Waiting Time (W) | The average time a customer or job spends waiting in the queue. |
System Length (L<sub>s</sub>) | The average number of customers or jobs in the system (including those being served). |
System Time (W<sub>s</sub>) | The average time a customer or job spends in the system (waiting and being served). |
Utilization (ρ) | The ratio of arrival rate to service rate (ρ = λ/μ). Represents the proportion of time the server is busy. |
Queue Discipline | The rule determining the order in which customers or jobs are served (e.g., FIFO, LIFO, priority). |
Let's dive deeper into the key aspects of queuing theory, starting with its fundamental concepts and progressing to practical applications.
Understanding the Basic Elements of a Queuing System
A queuing system can be generally represented by the Kendall's notation: A/B/c.
- A: Describes the arrival process (e.g., M for Markovian or Poisson arrival, D for deterministic arrival, G for general arrival). This indicates the probability distribution governing the time between arrivals.
- B: Describes the service process (e.g., M for Markovian or exponential service, D for deterministic service, G for general service). This indicates the probability distribution governing the service time.
- c: Represents the number of servers in the system.
Common Queuing Models:
The simplest and most widely used model is the M/M/1 queue, where arrivals follow a Poisson process (M), service times follow an exponential distribution (M), and there is only one server (1). Other models, such as M/M/c (multiple servers), M/G/1 (general service time), and G/G/1 (general arrival and service time) exist to cater to more complex scenarios. The complexity of the model increases with the complexity of the arrival and service processes.
Analyzing a Queuing System:
Analyzing a queuing system involves calculating key performance indicators (KPIs) such as:
- Average queue length (L): This tells us the average number of customers waiting in the queue.
- Average waiting time (W): This indicates the average time a customer spends waiting before being served.
- Average number of customers in the system (L<sub>s</sub>): This includes both those waiting and those being served.
- Average time in the system (W<sub>s</sub>): This is the total time a customer spends in the system, including waiting and service time.
- Server utilization (ρ): This shows how busy the server is. A utilization close to 1 indicates a heavily loaded system, potentially leading to long queues and waiting times.
These KPIs are crucial for understanding the efficiency and performance of the system. They can be calculated using various formulas derived from the specific queuing model being used. For instance, in an M/M/1 queue, the average queue length (L) is given by: L = ρ² / (1 - ρ), where ρ = λ/μ.
Real-World Applications of Queuing Theory
Queuing theory finds applications in a diverse range of fields:
- Telecommunications: Analyzing call center performance, optimizing network design, and managing data traffic.
- Healthcare: Improving patient flow in hospitals, optimizing emergency room operations, and managing appointment scheduling.
- Manufacturing: Optimizing production lines, managing inventory, and scheduling maintenance.
- Transportation: Analyzing traffic flow, optimizing airport operations, and designing public transportation systems.
- Computer Science: Managing computer networks, optimizing database systems, and scheduling processes in operating systems.
- Retail: Optimizing store layouts, managing checkout lines, and staffing levels.
Example: Optimizing a Call Center
Imagine a call center with an average arrival rate of 10 calls per minute and an average service time of 3 minutes per call. This translates to λ = 10 calls/minute and μ = 1/3 calls/minute. Using the M/M/1 model, the server utilization (ρ) is calculated as ρ = λ/μ = 10 / (1/3) = 30. This indicates an extremely overloaded system (ρ > 1), implying infinitely long queues and unacceptable waiting times. To improve performance, the call center could:
- Increase the number of servers: Adding more agents will reduce the load on each server, lowering waiting times.
- Improve service time: Training agents to handle calls more efficiently, implementing better call routing systems, or using self-service options can reduce service time.
- Implement a call-back system: This reduces the need for customers to remain on hold.
- Employ predictive dialing: This can help optimize call routing and reduce idle time for agents.
By applying queuing theory, the call center can analyze the impact of different strategies and choose the most effective solution to optimize their operations.
Exploring the Connection Between Customer Satisfaction and Queuing Theory
Customer satisfaction is directly impacted by waiting times. Long queues lead to frustration and negative experiences, potentially damaging brand reputation and customer loyalty. Queuing theory provides a quantitative framework for understanding this relationship. By predicting waiting times, organizations can proactively manage customer expectations and improve overall satisfaction. For example, a retailer could use queuing models to estimate wait times at checkout counters during peak hours and adjust staffing levels accordingly. They could also provide real-time queue information to customers, allowing them to manage their time more effectively. This transparency contributes significantly to a better customer experience.
Further Analysis of Queue Discipline
The queue discipline significantly affects the performance of a queuing system. Different disciplines lead to different waiting times and queue lengths. Common queue disciplines include:
- First-In, First-Out (FIFO): Customers are served in the order they arrive. This is the most common and often fairest approach.
- Last-In, First-Out (LIFO): Customers are served in the reverse order they arrive. This is less common and may lead to unfairness.
- Priority Queuing: Customers are prioritized based on certain criteria (e.g., urgency, importance). This requires a careful consideration of prioritization rules to avoid starvation of lower-priority customers.
Choosing the appropriate queue discipline depends on the specific context and objectives. For instance, a hospital emergency room might prioritize patients based on the severity of their condition, while a supermarket checkout might use FIFO to ensure fairness.
The following table summarizes different queue disciplines and their impact:
Queue Discipline | Advantages | Disadvantages | Suitable Applications |
---|---|---|---|
FIFO | Simple, fair, easy to implement | Can lead to long waiting times for all customers | Supermarkets, banks, general service counters |
LIFO | May prioritize urgent tasks | Can lead to unfairness and starvation of some customers | Stack-based systems, limited buffer situations |
Priority Queuing | Efficient for prioritizing urgent tasks | Can lead to starvation of low-priority customers | Hospitals (emergency rooms), task scheduling systems |
Shortest Job First | Minimizes average waiting time | Requires knowledge of service times | Computer systems (process scheduling), manufacturing |
Frequently Asked Questions (FAQ)
-
What is the difference between an M/M/1 and an M/M/c queue? An M/M/1 queue has a single server, while an M/M/c queue has multiple servers. The M/M/c model is more realistic for systems with multiple servers handling customer requests.
-
How can I determine the best queuing model for my system? The choice of model depends on the characteristics of your arrival and service processes. If arrivals and service times are approximately exponential, an M/M/1 or M/M/c model is suitable. For more complex scenarios with non-exponential distributions, more general models (e.g., G/G/1) may be needed.
-
What if my system has limited capacity? Queuing models can be extended to incorporate limited queue capacity. This means that once the queue reaches its maximum size, further arrivals are either blocked or lost.
-
How can I use queuing theory to improve my system's performance? By analyzing the KPIs of your queuing system (e.g., waiting times, queue lengths, server utilization), you can identify bottlenecks and areas for improvement. You can then experiment with different strategies (e.g., adding servers, improving service efficiency, implementing a different queue discipline) and assess their impact on performance using queuing models.
-
Is queuing theory only applicable to physical queues? No. Queuing theory applies to any system where entities (customers, tasks, packets) arrive for service, wait in a queue if necessary, and then are served. This includes virtual queues in computer systems and telecommunications networks.
-
What software tools can be used for queuing analysis? There are several software packages that can perform queuing analysis, including simulation software like Arena and AnyLogic, and statistical software packages such as R and MATLAB.
Practical Tips for Applying Queuing Theory
-
Identify your arrival and service processes: Determine the probability distributions that best describe the arrival and service times in your system.
-
Choose an appropriate queuing model: Select a model that accurately reflects the characteristics of your system (number of servers, arrival and service distributions, queue capacity).
-
Calculate key performance indicators (KPIs): Compute KPIs such as average waiting time, queue length, and server utilization to assess the performance of your system.
-
Identify bottlenecks: Determine the parts of your system that are contributing most to delays and congestion.
-
Implement improvements: Based on your analysis, implement strategies to improve performance (e.g., adding servers, improving service times, implementing a different queue discipline).
-
Monitor your system: Continuously monitor your system’s performance to track the impact of your improvements and identify further areas for optimization.
-
Validate your model: Compare the results predicted by your model with actual data from your system to ensure the model's accuracy.
-
Consider human factors: When designing systems that involve human interaction, remember to account for human behavior and limitations.
Final Conclusion
Queuing theory provides a powerful and versatile framework for analyzing and optimizing systems facing congestion. Its applications extend across a wide range of fields, impacting efficiency, productivity, and customer satisfaction. By understanding the fundamental elements of queuing theory and applying its principles effectively, organizations can significantly enhance the performance of their systems and achieve greater operational excellence. The ongoing development and refinement of queuing models ensure its continued relevance in a constantly evolving technological landscape. Further exploration of advanced queuing models and simulation techniques will undoubtedly unveil further insights and applications of this crucial analytical tool.
Latest Posts
Related Post
Thank you for visiting our website which covers about Queuing Theory Definition Elements And Example . We hope the information provided has been useful to you. Feel free to contact us if you have any questions or need further assistance. See you next time and don't miss to bookmark.