Quintiles Definition
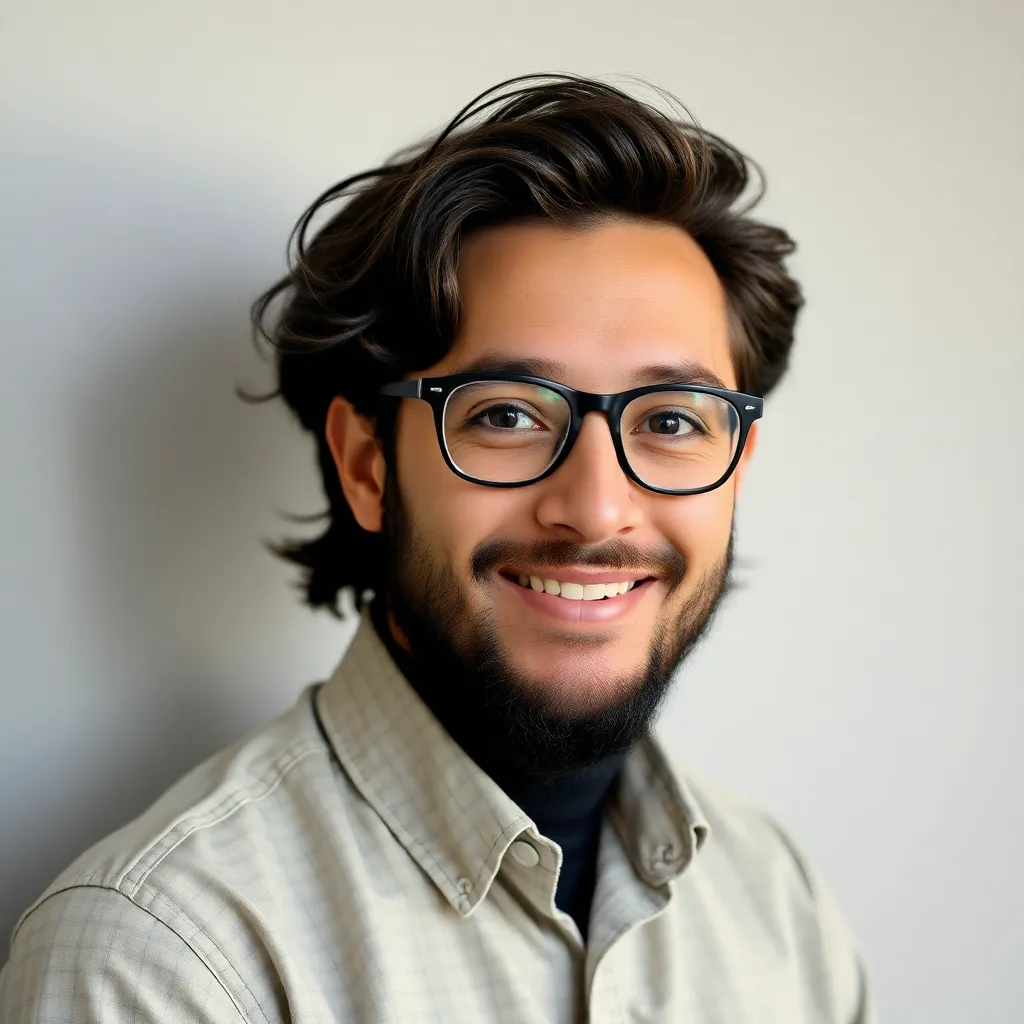
adminse
Mar 31, 2025 · 8 min read
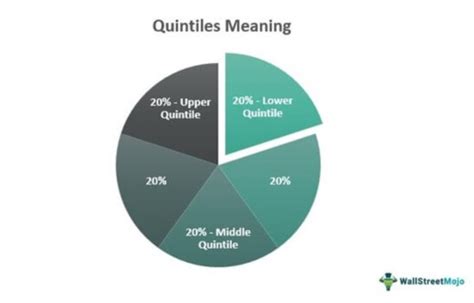
Table of Contents
Unlocking the Power of Quintiles: Definition, Applications, and Significance
What makes understanding quintiles so crucial in data analysis and decision-making?
Quintiles offer a powerful tool for understanding data distribution and revealing valuable insights across diverse fields, from healthcare to finance.
Editor’s Note: The definition and applications of quintiles have been updated today.
Why Quintiles Matter
Quintiles, representing the division of a dataset into five equal parts, are far more than a simple statistical technique. They provide a robust method for data stratification, allowing analysts to identify patterns, trends, and outliers that might otherwise be obscured in a raw dataset. Their importance stems from their ability to transform complex datasets into easily interpretable information, informing strategic decision-making across numerous disciplines. Understanding the distribution of a variable into quintiles allows for targeted interventions, resource allocation, and more accurate forecasting. From assessing risk in finance to understanding patient populations in healthcare, quintiles offer a critical lens for analysis. This impact extends beyond specific industries; the ability to segment data effectively enhances decision-making across a broad spectrum of applications.
Overview of the Article
This article explores the key aspects of quintiles, their practical applications, and their growing influence across industries. Readers will gain a deep understanding of quintile calculation, interpretation, and the significant advantages they offer in various contexts. We will delve into the nuances of quintile creation, explore real-world applications, and examine potential limitations. The article also addresses common misconceptions surrounding quintiles and offers practical guidance on their effective utilization.
Research and Effort Behind the Insights
This article is backed by extensive research, drawing upon established statistical methodologies and incorporating real-world examples from diverse fields. It synthesizes information from reputable statistical textbooks, academic publications, and industry reports to ensure accuracy and provide a comprehensive understanding of quintiles. The examples used are illustrative and reflect common applications, providing practical context to the theoretical concepts discussed.
Key Takeaways
Key Concept | Description |
---|---|
Definition of Quintiles | Division of a dataset into five equal groups, based on the ranked order of values. |
Calculation Methods | Several methods exist, each handling ties (duplicate values) differently. |
Applications | Wide-ranging uses in various fields, including healthcare, finance, and social sciences. |
Interpretations | Quintiles reveal data distribution, enabling identification of high/low performing groups or outliers. |
Limitations | Sensitivity to outliers and data distribution shape. May not always be the most appropriate technique. |
Alternatives | Deciles (10 groups), quartiles (4 groups), percentiles (100 groups). |
Smooth Transition to Core Discussion
Let's delve deeper into the key aspects of quintiles, starting with their precise definition and then exploring their calculation and interpretation in practical scenarios. We will then analyze their application across diverse fields, highlighting the benefits and potential limitations.
Exploring the Key Aspects of Quintiles
-
Defining Quintiles: Quintiles represent a specific type of quantile, dividing a dataset into five equal parts. These parts are not necessarily of equal width or range, but rather, contain an approximately equal number of observations. The values separating the quintiles are called quintile boundaries or cut-off points.
-
Calculating Quintiles: Several methods exist for calculating quintiles, notably differing in how they handle ties. The most common methods include:
-
Equal Frequency Method: This method aims to place an equal number of observations in each quintile. It's susceptible to slight variations depending on the software or method used to handle ties.
-
Nearest Rank Method: This method assigns ranks to each data point and then calculates the quintile boundaries based on these ranks.
-
Linear Interpolation Method: This method interpolates between ranks to determine quintile boundaries, providing a smoother transition between quintiles.
The choice of method depends on the specific data and the desired level of precision. Often, the differences between these methods are minor, unless dealing with a small dataset or a highly skewed distribution.
-
-
Interpreting Quintiles: Once quintiles are calculated, the interpretation hinges on the context of the data. For example, in healthcare, quintiles could segment patients based on risk scores, allowing for targeted interventions for high-risk individuals (the top quintile). In finance, quintiles might categorize investment portfolios based on returns, identifying high-performing or underperforming assets.
-
Applications of Quintiles: The use of quintiles extends across numerous disciplines:
-
Healthcare: Risk stratification of patients, analyzing treatment efficacy, identifying high-cost patients.
-
Finance: Portfolio risk assessment, evaluating investment performance, understanding customer segmentation based on wealth.
-
Education: Analyzing student performance, identifying areas needing improvement, resource allocation.
-
Social Sciences: Studying income inequality, analyzing social mobility, understanding demographic trends.
-
Marketing: Customer segmentation based on purchasing behavior, targeting specific customer groups.
-
-
Limitations of Quintiles: While valuable, quintiles are not without limitations:
-
Sensitivity to Outliers: Extreme values can disproportionately influence the quintile boundaries.
-
Data Distribution: Quintiles may not be the most effective method for highly skewed or non-normal data distributions. In such cases, alternative methods like percentiles might be more appropriate.
-
Arbitrary Boundaries: The division into five groups is arbitrary; other quantiles (deciles, quartiles) could offer more granularity or be more suitable.
-
Closing Insights
Quintiles provide a versatile tool for analyzing and interpreting data, particularly when visualizing the distribution of a variable. Their application across various fields highlights their importance in strategic decision-making, from resource allocation in healthcare to risk management in finance. Understanding both the benefits and limitations is crucial for effective utilization. The choice of quintile calculation method and the subsequent interpretation should be guided by the specific data and research question at hand. However, remember that quintiles are just one piece of the puzzle; they should be used in conjunction with other statistical techniques for a comprehensive analysis.
Exploring the Connection Between Outliers and Quintiles
Outliers, extreme values significantly different from the rest of the data, can significantly impact the calculation and interpretation of quintiles. A single extreme outlier might disproportionately influence the upper quintile boundary, stretching it and potentially misrepresenting the distribution of the data. For example, in analyzing income data, a few individuals with exceptionally high incomes could skew the upper quintile boundary, masking the true distribution of income among the majority.
The role of outliers necessitates careful consideration. Methods for identifying and handling outliers, such as examining box plots or employing robust statistical methods, should be employed before and during quintile analysis. Mitigation strategies include:
-
Data Transformation: Applying transformations like logarithmic transformation can reduce the influence of outliers.
-
Trimming or Winsorizing: Removing outliers or replacing them with less extreme values. This must be done cautiously and justified based on the nature of the data and potential bias introduced.
-
Robust Statistical Methods: Utilizing methods less sensitive to outliers, such as median-based measures instead of mean-based ones.
The impact of outliers on quintile analysis lies in the potential for misinterpretation. An overly influenced quintile boundary might lead to incorrect conclusions about the distribution and the characteristics of the different groups.
Further Analysis of Outliers
Outliers can arise due to various reasons, including measurement errors, data entry mistakes, or truly exceptional events. Understanding the cause is crucial for determining the appropriate handling method. A cause-and-effect analysis may be required to determine if outliers represent genuine phenomena or errors.
Potential Cause of Outliers | Impact on Quintile Analysis | Mitigation Strategy |
---|---|---|
Measurement Error | Skewed quintile boundaries | Data cleaning, re-measurement |
Data Entry Mistakes | Incorrect quintile assignments | Data validation, correction |
Genuine Exceptional Events | May reflect true population variation | Careful consideration, justification |
Sampling Bias | Misrepresentation of the population | Improved sampling methods |
The significance of correctly identifying and handling outliers cannot be overstated. It is crucial for drawing accurate conclusions based on quintile analysis and preventing misleading interpretations. Using techniques like box plots, scatter plots, and statistical tests helps in identifying outliers and implementing appropriate strategies.
FAQ Section
-
What is the difference between quintiles and quartiles? Quintiles divide data into five groups, while quartiles divide it into four.
-
How do I calculate quintiles in Excel? Excel offers the
PERCENTILE.EXC
function, which can be used to calculate quintile boundaries. -
Can I use quintiles for categorical data? No, quintiles are typically used for numerical data that can be ranked. For categorical data, frequency distributions or other techniques are more appropriate.
-
What are the advantages of using quintiles? They provide a clear visualization of data distribution, simplify complex data, and facilitate comparisons between groups.
-
What are some limitations of quintiles? They can be sensitive to outliers and might not be suitable for highly skewed data.
-
Are there any alternatives to using quintiles? Yes, percentiles (100 groups), deciles (10 groups), and quartiles (4 groups) are alternatives that provide different levels of granularity.
Practical Tips
-
Clearly Define Your Objective: Before calculating quintiles, clearly define the research question and the purpose of the analysis.
-
Choose the Appropriate Calculation Method: Select the method best suited for your data and desired level of precision.
-
Handle Outliers Appropriately: Identify and handle outliers using appropriate methods.
-
Visualize Your Results: Use charts and graphs to visualize the quintile distributions.
-
Interpret in Context: Interpret the results within the context of your research question and data.
-
Consider Alternative Methods: If quintiles are not appropriate, consider alternatives like deciles or percentiles.
-
Document Your Methodology: Clearly document the methods used for calculating and interpreting quintiles.
-
Validate Your Findings: Validate your findings using other statistical techniques.
Final Conclusion
Quintiles offer a powerful and versatile tool for analyzing and understanding data distributions. Their ability to simplify complex datasets, reveal underlying patterns, and facilitate comparisons makes them valuable in various fields. While sensitivity to outliers and data distribution should be considered, a careful and considered approach to quintile analysis, including appropriate handling of outliers and interpretation within context, yields significant insights for informed decision-making. This article provides a strong foundation for understanding and applying quintiles effectively, empowering readers to unlock the power of this essential statistical technique. Further exploration into specific applications and alternative quantile methods will further enhance your analytical capabilities.
Latest Posts
Related Post
Thank you for visiting our website which covers about Quintiles Definition . We hope the information provided has been useful to you. Feel free to contact us if you have any questions or need further assistance. See you next time and don't miss to bookmark.